Coding the Way to Better Patient Outcomes: Machine Learning at Cigna
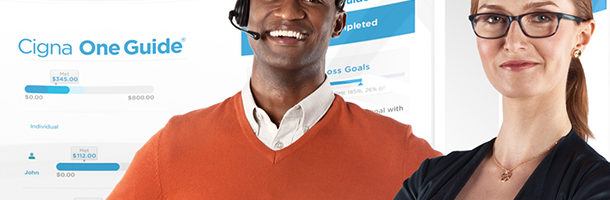
American health insurer Cigna must balance the benefits of machine learning with the internal challenges that arise during implementation and the external ethical implications inherent to the healthcare industry.
(Image Citation – https://www.cigna.com/guideme)
A Changing Landscape
As the healthcare industry in the United States shifts toward a pay-for-performance model, many insurers, like Cigna, are embracing patient-centric approaches to care compensation.[1] For Cigna, the fourth largest health insurer in the United States, data has become a key competitive asset in responding to this new healthcare landscape.[2] To actually tap into the value of this data, Cigna has strategically made investments in machine learning.[3]
Machine Learning brings both Opportunities and Challenges
As part of this investment, Cigna faces the challenge of reevaluating its management of product development within the company. The incorporation of machine learning places new requirements on Cigna to not only increase investment in data infrastructure and talent, but also to rethink the way in which the company engages and interacts with patients[4]. Specifically, Cigna faces three key questions as it works to leverage the benefits of machine learning:
- Can Cigna actually deliver on predicting patient outcomes? Machine learning is inherently complex, and implementation is a huge factor in overall success.[5]
- Is Cigna engaged enough with its patient and provider populations to deliver machine learning insights? Regardless of how great the insights are, patients and providers need to be aware of, and have easy access to, these insights.
- Will a new patient-centric strategy be difficult for Cigna to implement? Traditionally, the company has focused on forging B2B relationships.[6]
To address these key questions, Cigna’s short-term implementation of Machine Learning has largely focused on their existing historical data set.[7] Based on this historical information, Cigna’s machine learning algorithms have had success in predicting when patient issues will arise and in helping providers assess the most appropriate treatments.[8] Cigna has also rolled out a patient facing application call OneGuide, which engages with patients and surfaces insights during the important moments of care.[9] Through their research, data scientists at Cigna have found that increased patient engagement results in better health outcomes for patients, and that the timing of these engagements is critically important.[10] With over 10% of Cigna insured patients using the app as of 2017, Cigna is seeing some significant traction.[11]
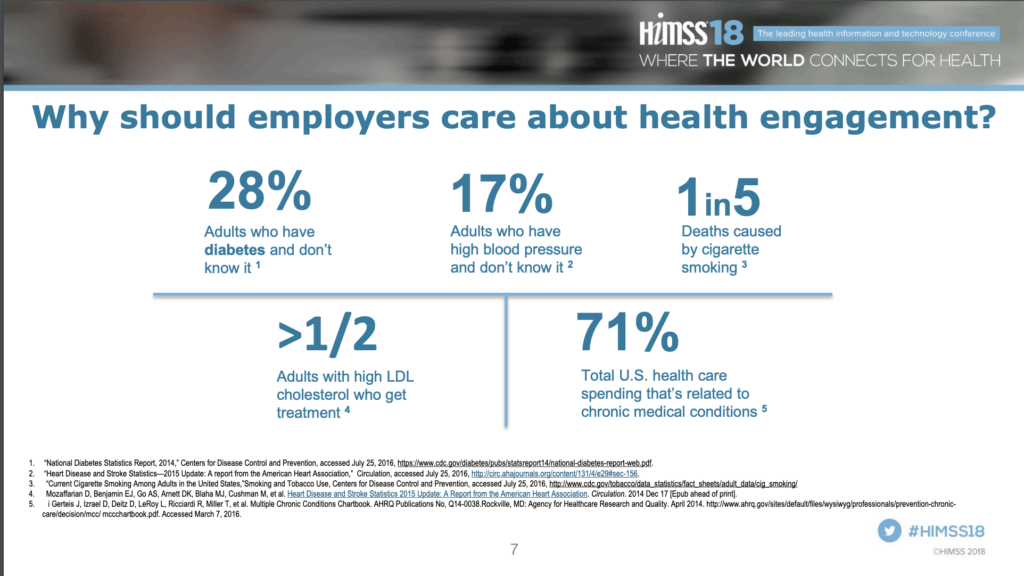
Longer term, Cigna wants to create more complex machine learning techniques in the areas of chronic disease prevention, claims fraud, and wearables.[12] To do this, Cigna will need to leverage more data, likely from both internal and external sources. This poses additional risks, as third-party data is often more difficult integrate into existing models, challenging to acquire, and hard to verify in terms of accuracy.[13] Cigna has also been making strategic investments in startups who are also focused on using machine learning data to drive better patient outcomes.[14]
Next Steps for Cigna
While Cigna has been successful in addressing the aforementioned challenges, the company will need to focus on deeper customer engagement in order to ensure continued success in the near term. The initial traction of OneGuide is very promising, and Cigna should double down on their investment in patient facing applications and work to drive more patients to the platform. Gaining a stronger foothold here will make it easier for Cigna to surface future machine learning insights to customers, while also creating inroads for Cigna to become more involved in the overall care of patients.
It is also important for Cigna to consider the ethical challenges that can arise through the use of machine learning. According to a study that appeared in the New England Journal of Medicine, “Algorithms introduced in non-medical fields have already been shown to make problematic decisions that reflect biases inherent in the data used to train them.”[15] Especially for an insurer like Cigna, who has access to a plethora of patient data and is actively working to help influence patient decisions, awareness of ethical biases and constructing safeguards to prevent them will be extremely important.
Longer term, Cigna should consider growing their machine learning investment via acquisition. With a wide range of promising startups specifically focusing on machine learning and patient outcomes, Cigna could attain a fast mover advantage by acquiring some of these companies.[16] Additionally, Cigna should focus on not only expanding its data set to incorporate more outside sources, but in structuring and cleaning-up this data. Healthcare today is plagued with data issues that can largely be blamed on the lack of interoperability between platforms.[17] Cigna has an opportunity here to not only improve input data for its own machine learning models, but for the healthcare industry as a whole.[18]
The Big Question
Interestingly, Cigna’s focus on machine learning and its initial success in implementing it has surfaced unique ethical issues. What responsibility does Cigna have in helping the entire U.S healthcare industry improve patient outcomes, and how should they balance this responsibility with the need to maintain a competitive edge?
(Word Count – 789)
[1] “What is Pay for Performance in Healthcare?,” NEJM Catalyst, March 1, 2018, https://catalyst.nejm.org/pay-for-performance-in-healthcare/, accessed November 2018.
[2] “America’s Biggest Health Insurance Providers,” https://www.forbes.com/pictures/fefi45ejdih/4-cigna/#20e83885779b, accessed November 2018.
[3] Cigna, 2017 Annual Report, p.45, https://www.cigna.com/assets/docs/annual-reports-and-proxy-statements/cigna-2017-annual-report.pdf?WT.z_nav=about-us%2Finvestors%2Fannual-reports-and-proxy-statements%3Blink-List%3BAnnual%20Reports%3B2017%20Annual%20Report, accessed November 2018.
[4] Cigna, 2017 Annual Report, p.45.
[5] “The Challenges and Opportunities of Implementing AI in Healthcare”, PokitDok, July 31, 2018, https://blog.pokitdok.com/ai-in-healthcare/, accessed November 2018.
[6] Jonah Comstock, “For Cigna, United Healthcare, digital innovation is all about the customer,” mobi health news, October 6, 2017, https://www.mobihealthnews.com/content/cigna-unitedhealthcare-digital-innovation-all-about-customer, accessed November 2018.
[7] Mark Boxer, “Cigna uses artificial intelligence to sift through big data ‘noise’,” Managed Healthcare Executive, February, 8, 2018, http://www.managedhealthcareexecutive.com/mhe-articles/cigna-uses-artificial-intelligence-sift-through-big-data-noise, accessed November 2018.
[8] Mark Boxer, “Cigna uses artificial intelligence to sift through big data ‘noise’”.
[9] Jonah Comstock, “For Cigna, United Healthcare, digital innovation is all about the customer”.
[10] Christer Johnson and Doug Melton, “Machine learning and Big Data to Drive Better Health Outcomes,” PowerPoint Presentation, March 5, 2018, HIMSS18, Las Vegas, NV, http://365.himss.org/sites/himss365/files/365/handouts/550235514/handout-20180308143157-275.pdf, accessed November 2018.
[11] Jonah Comstock, “For Cigna, United Healthcare, digital innovation is all about the customer”.
[12] Mark Boxer, “Cigna uses artificial intelligence to sift through big data ‘noise’”.
[13] Donna Marbury, “Six Healthcare Interoperability Barriers to Watch”, Managed Healthcare Executive, November 1, 2017, http://www.managedhealthcareexecutive.com/business-strategy/six-healthcare-interoperability-barriers-watch, accessed November 2018.
[14] Erin Dietsche, “Prognos raises $20,5 million from investors including Cigna, Merck Global, Health Innovation Fund,” MedCity News, December 4, 2017, https://medcitynews.com/2017/12/prognos-investors/, accessed November 2018.
[15] Char DS, Shah NH, Magnus D. Implementing Machine Learning in Health Care – Addressing Ethical Challenges. N Engl J Med. 2018;378(11):981-983.
[16] Louis Columbus, “25 Machine Learning Startups to Watch in 2018,” Forbes, August 26, 2018, https://www.forbes.com/sites/louiscolumbus/2018/08/26/25-machine-learning-startups-to-watch-in-2018/#3a6d2b776f99, accessed November 2018.
[17] Amelia Coleman, “Solving the Interoperability Crisis in Healthcare with Consumer Tech, Becker’s Health IT & CIO Report, June 22, 2017, https://www.beckershospitalreview.com/healthcare-information-technology/solving-the-interoperability-crisis-in-healthcare-with-consumer-tech.html, accessed November 2018
[18] Matt McElheny,“Facing Data Integration Demands”, Healthcare IT News, April 1, 2015, https://www.healthcareitnews.com/blog/facing-data-integration-demands, accessed November 2018.
Predicting patient outcomes has been a never ending struggle in health-services research. Predictive models (such as the APACHE score, readmission calculators, cardiovascular risk scores) have become increasingly complex as access to data increases. However, you are right that data silos prevent the translation of these models into actionable intelligence. Patients and clinicians rarely see health predictions in real time to take action that can improve health outcomes. I agree that Cigna and other aggregators of health data across the ecosystem have a responsibility to society to use that data responsibly to help people.
Very interesting. I learned how to bill insurance companies, including Cigna, over the course of the past year while working at an ambulance company and it was among the most complex, non-intuitive tasks that I’ve had to learn. I would be curious if the machine learning that Cigna is investing in could be applied to fixing the company’s claim reimbursement process. Aside from the billing / claim reimbursement side of the business it sounds like the machine learning that Cigna is investing in will hopefully benefit the firms customers. Does the increased cost base cause an up-tick in the cost of acquiring insurance for consumers? Interesting and informative essay – really enjoyed reading it.
I’m glad to see that Cigna is thinking of ways to use their machine learning predictive capabilities to help patients, becoming patient and outcome focused. However, I have trouble believing that these methods are being used entirely (or even primarily) in such ethical ways. As we’ve learned in our finance class, riskier investments require a higher rate of return. If an insurer is able to predict future health problems using AI they may deem a customer as more “risky”. This risk perception could allow them to justify increasing insurance prices for high risk patients, or refusing to insure the patient altogether. Further more, I wonder about the ethics of making such a decision. From a financial standpoint, if Cigna has the ability to detect that a consumer has a “NPV < 0" due to their risk profile, is it ethical for them to choose not to ensure them (or to hike their price up)?
Interesting concept – this seems like a natural extension for machine learning and hopefully can have a real impact on people’s lives. I’m an optimist, and think both goals can be accomplished – Cigna should be able to maintain a competitive edge while also helping patients. Data should be used in tandem with human contact/judgement to ensure the best outcome for the patient. I do like the idea of health data permeated all aspects of life to help with data collection. I think a lot of people would be willing to wear watches etc. for the greater good.
This is very interesting; thank you for sharing. I’m wondering what types of data (“variety”) OneGuide currently collects and to what extent the data collection process is passive versus active. In addition to the clinical and physiologic data that Cigna has, I wonder if they’re making similar investments into patient-reported outcomes and how they see the role of technologies such as digital phenotyping (moment-to-moment quantification of patient health states and well-being) informing critical decisions such as early intervention, prevention and population health.
Thank you for a great read. Nowadays it seems as if every healthcare company with access to data is trying to create data guided recommendations. A challenge that Cigna may have is ensuring its tools that it may create or recommendations it may find are largely based upon retrospective data. I wonder if they can find a way to run rigorous trials within their patient populations testing the ability of certain recommendations to effect morbidity or mortality. Another concern would be that Cigna must enroll representative patient populations – or else it may end up creating a new set of recommendations for people that have relatively better access to care and can therefore further health inequity.